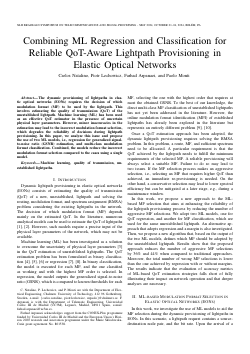
Combining ML Regression and Classification for Reliable QoT-Aware Lightpath Provisioning in Elastic Optical Networks
Carlos Natalino, Piotr Lechowicz, Farhad Arpanaei, Paolo Monti
DOI: 10.14209/sbrt.2024.1571036652
Evento: XLII Simpósio Brasileiro de Telecomunicações e Processamento de Sinais (SBrT2024)
Keywords: Machine learning Artificial intelligence Quality of transmission Elastic optical networks
Abstract
The dynamic provisioning of lightpaths in elastic optical networks (EONs) requires the decision of which modulation format (MF) to be used by the lightpath. This involves estimating the quality of transmission (QoT) of the unestablished lightpath. Machine learning (ML) has been used as an effective QoT estimator in the presence of uncertain physical layer parameters. However, minor inaccuracies in the estimation may lead to the incorrect modulation format selection, which degrades the reliability of decisions during lightpath provisioning. In this paper, we analyze this issue and propose the use of two ML models, i.e., regression for generalized signal- to-noise ratio (GSNR) estimation, and multi-class modulation format classification. Combined, the models reduce the incorrect modulation format selection compared to the cases using a single model.Download