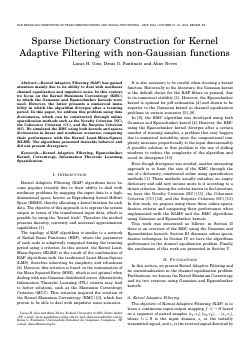
Sparse Dictionary Construction for Kernel Adaptive Filtering with non-Gaussian Functions
Lucas Gois, Denis Fantinato, Aline Neves
DOI: 10.14209/sbrt.2024.1571036483
Evento: XLII Simpósio Brasileiro de Telecomunicações e Processamento de Sinais (SBrT2024)
Keywords: Kernel Adaptive Filtering Epanechnikov Kernel Correntropy Sparsification
Abstract
Kernel Adaptive Filtering (KAF) has gained attention mainly due to its ability to deal with nonlinear channel equalization and impulsive noise. In this context, we focus on the Kernel Maximum Correntropy (KMC), in which the Gaussian and Epanechnikov kernels were used. However, the latter presents a numerical instability in which the algorithm diverges after a training period. In this paper, we address this problem using data dictionaries, which can be constructed through online sparsification methods such as the Novelty Criterion (NC), the Coherence Criterion (CC), and the Surprise Criterion (SC). We simulated the KMC using both kernels and sparse dictionaries in linear and nonlinear scenarios, comparing their performance with the Kernel Least-Mean-Square (KLMS). The algorithms presented desirable behavior and did not present divergence.Download