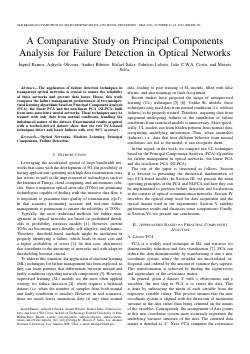
A Comparative Study on Principal Components Analysis for Failure Detection in Optical Networks
Ingrid L. F. M. Ramos, Adryele C. Oliveira, Andrei Nogueira Ribeiro, Rafael Sales, Fabrício Lobato, Joao Weyl Costa, Moisés Felipe Silva
DOI: 10.14209/sbrt.2024.1571036264
Evento: XLII Simpósio Brasileiro de Telecomunicações e Processamento de Sinais (SBrT2024)
Keywords: Optical Networks Machine Learning Principal Components Failure Detection
Abstract
The application of failure detection techniques in transparent optical networks is crucial to ensure the reliability of these networks and prevent data losses. Hence, this work compares the failure management performances of two unsupervised learning algorithms based on Principal Component Analysis (PCA): the linear PCA and the non-linear PCA (NLPCA) built from auto-associative neural networks. These techniques may be trained with only data from normal conditions, handling the imbalanced nature of the dataset. Experimental results acquired with a testbed-derived dataset show that the two PCA-based techniques detect and locate failures with over 90\% accuracy.Download