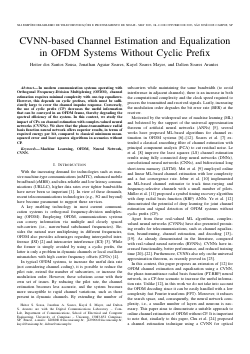
CVNN-based Channel Estimation and Equalization in OFDM Systems Without Cyclic Prefix
Heitor dos Santos Sousa, Jonathan Aguiar Soares, Kayol S. Mayer, Dalton Arantes
DOI: 10.14209/sbrt.2023.1570923809
Evento: XLI Simpósio Brasileiro de Telecomunicações e Processamento de Sinais (SBrT2023)
Keywords: Machine Learning OFDM Neural Network CVNN
Abstract
In modern communication systems operating with Orthogonal Frequency-Division Multiplexing (OFDM), channel estimation requires minimal complexity with one-tap equalizers. However, this depends on cyclic prefixes, which must be sufficiently large to cover the channel impulse response. Conversely, the use of cyclic prefix (CP) decreases the useful information that can be conveyed in an OFDM frame, thereby degrading the spectral efficiency of the system. In this context, we study the impact of CPs on channel estimation with complex-valued neural networks (CVNNs). We show that the phase-transmittance radial basis function neural network offers superior results, in terms of required energy per bit, compared to classical minimum mean-squared error and least squares algorithms in scenarios without CP.Download