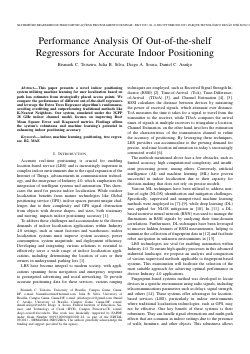
Performance Analysis Of Out-of-the-shelf Regressors for Accurate Indoor Positioning
Bismark C Teixeira, Julia B Silva, Diego Aguiar Sousa, Daniel Araújo
DOI: 10.14209/sbrt.2023.1570923594
Evento: XLI Simpósio Brasileiro de Telecomunicações e Processamento de Sinais (SBrT2023)
Keywords:
Abstract
This paper presents a novel indoor positioning system utilizing machine learning for user localization based on path loss estimates from strategically placed access points. We compare the performance of different out-of-the-shelf regressors and leverage the Extra Trees Regressor algorithm's randomness, avoiding overfitting and outperforming traditional methods like K-Nearest Neighbors. Our system, simulated under the 3GPP 28 GHz indoor channel model, focuses on improving Root Mean Square Error and R-squared metrics. Findings affirm the system's robustness and machine learning's potential in enhancing indoor positioning accuracy.Download