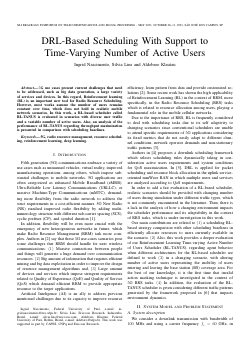
DRL-Based Scheduling With Support to Time-Varying Number of Active Users
Ingrid Nascimento, Silvia Lins, Aldebaro Klautau
DOI: 10.14209/sbrt.2023.1570923579
Evento: XLI Simpósio Brasileiro de Telecomunicações e Processamento de Sinais (SBrT2023)
Keywords: 5G Radio Resource Management Resource Scheduling Reinforcement Learning
Abstract
5G use cases present current challenges that needs to be addressed such as, big data generation, large variety of services and devices. In this regard, Reinforcement learning (RL) is an important new tool for Radio Resource Scheduling. However, most works assume the number of users remains constant over time, which does not hold in realistic mobile network scenarios. In this work, an RL-based scheduler called RL-TANUS is evaluated in scenarios with diverse user traffic and with a variable number of active users. Also, an analysis of the performance of RL-TANUS regarding throughput maximization is presented in comparison with scheduling baselines.Download