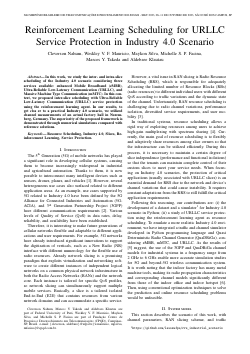
Reinforcement Learning Scheduling for URLLC Service Protection in Industry 4.0 Scenario
Cleverson Nahum, Weskley Maurício, Maykon Silva, Michelle Soares Pereira Facina, Marcos Takeda, Aldebaro Klautau
DOI: 10.14209/sbrt.2023.1570922913
Evento: XLI Simpósio Brasileiro de Telecomunicações e Processamento de Sinais (SBrT2023)
Keywords: Resource Scheduling Industry 4.0 Slices Reinforcement Learning
Abstract
In this work, we study the inter- and intra-slice scheduling of the Industry 4.0 scenario considering three services available: enhanced Mobile Broadband (eMBB), Ultra-Reliable Low-Latency Communication (URLLC), and Massive Machine Type Communication (mMTC). In this context, we proposed inter-slice scheduling with Ultra-Reliable Low-Latency Communication (URLLC) service protection using the reinforcement learning agent. In our results, to get closer to a practical Industry 4.0 scenario, we utilized channel measurements of an actual factory hall in Nuremberg, Germany. The superiority of the proposed framework is demonstrated through numerical simulations compared with reference solutions.Download