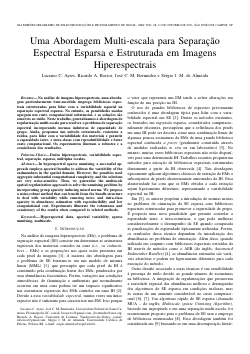
Uma Abordagem Multi-escala para Separação Espectral Esparsa e Estruturada em Imagens Hiperespectrais
Luciano Carvalho Ayres, Ricardo Augusto Borsoi, Jose Carlos Moreira Bermudez, Sérgio José Melo de Almeida
DOI: 10.14209/sbrt.2023.1570916644
Evento: XLI Simpósio Brasileiro de Telecomunicações e Processamento de Sinais (SBrT2023)
Keywords: dados hiperespectrais variabilidade espectral estruturas de endmembers separação esparsa
Abstract
In hyperspectral sparse unmixing, a successful approach employs spectral bundles to address the variability of the endmembers in the spatial domain. However, the penalties used aggregate substantial computational complexity, and the solutions are very noise-sensitive. Thus, we generalize the multiscale spatial regularization approach to solve the unmixing problem by incorporating group sparsity inducing mixed norms. We propose a noise-robust method that can benefit from the bundle structure to deal with variability while ensuring inter- and intra-class sparsity in abundance estimation with reproducibility and low computational cost. Experiments illustrate the robustness and consistency of the results when compared to related methods.Download