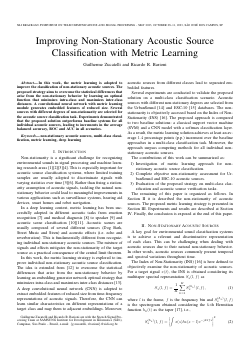
Improving Non-Stationary Acoustic Source Classification with Metric Learning
Guilherme Zucatelli, Ricardo Rossiter Barioni
DOI: 10.14209/sbrt.2023.1570915761
Evento: XLI Simpósio Brasileiro de Telecomunicações e Processamento de Sinais (SBrT2023)
Keywords: non-stationary acoustic sources multi-class classification metric learning
Abstract
In this work, the metric learning is adopted to improve the classification of non-stationary acoustic sources. The proposed strategy aims to overcome the statistical differences that arise from the non-stationary behavior by learning an optimal function that minimizes intra-class and maximizes inter-class distances. A convolutional neural network with metric learning module generates embedded features of reduced size. Several sources with different degrees of non-stationarity are selected for the acoustic source classification task. Experiments demonstrated that the proposed solution outperforms baseline systems for all individual acoustic sources, leading to increments in the average balanced accuracy, ROC and AUC in all scenarios.Download