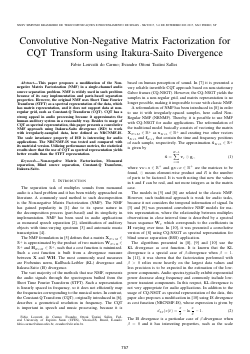
Convolutive Non-Negative Matrix Factorization for CQT Transform using Itakura-Saito Divergence
Fabio Louvatti do Carmo, Evandro Ottoni Teatini Salles
DOI: 10.14209/sbrt.2017.29
Evento: XXXV Simpósio Brasileiro de Telecomunicações e Processamento de Sinais (SBrT2017)
Keywords: Non-negative Matrix Factorization Monaural separation Blind source separation Constant-Q Transform Itakura-Saito
Abstract
This paper proposes a modification of the Nonnegative Matrix Factorization (NMF) in a single-channel audio source separation problem. NMF is widely used in such problem because of its easy implementation and parts-based separation properties. However, the original NMF uses Short Time Fourier Transform (STFT) as a spectral representation of the data, which has matrix representation, and it does not support data at nonregular grid, such as Constant-Q Transform (CQT). CQT has a strong appeal in audio processing because it approximates the human auditory system in a reasonably way. Besides to usage of CQT as spectral representation, this paper presents a convolutive NMF approach using Itakura-Saito divergence (ISD) to work with irregularly-sampled data, here defined as NRCNMF-IS. The scale invariance property of ISD is interesting for audio applications. The NRCNMF-IS was tested and compared with its matricial version. Utilizing performance metrics, the statistical results show that the use of CQT as spectral representation yields better results than the STFT representation.Download