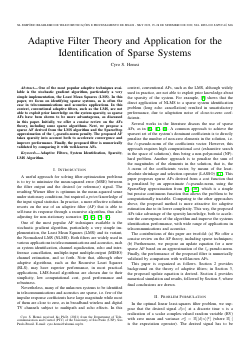
Adaptive Filter Theory and Application for the Identification of Sparse Systems
Cyro S. Hemsi
DOI: 10.14209/sbrt.2022.1570821925
Evento: XL Simpósio Brasileiro de Telecomunicações e Processamento de Sinais (SBrT2022)
Keywords: Adaptive Filters Sparsity System Identification
Abstract
One of the most popular adaptive algorithms available is the stochastic gradient algorithm, particularly a very simple implementation known as the least-mean-square (LMS) algorithm. Initially, this work offers a concise review of the adaptive filter (AF) theory, including sparse techniques. We then focused on identifying sparse channels, as is often the case in telecommunications and acoustics applications. In this case, conventional AFs, such as the LMS do not exploit prior knowledge of sparsity, therefore, sparse AFs have been shown to be more advantageous. This paper proposes sparse LMS-based AFs, derived from a simple approximation of the l_0-pseudo-norm penalty. These filters have low computational cost and are able to take advantage of channel sparsity, both to accelerate the convergence of estimates and improve the filter performance. Finally, the performance of the proposed methods is numerically validated by comparing them with well-known AFs.Download