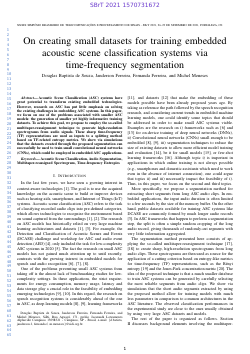
On creating small datasets for training embedded acoustic scene classification systems via time-frequency segmentation
Douglas Baptista de Souza, Janderson Ferreira, Fernanda Ferreira, Michel Meneses
DOI: 10.14209/sbrt.2021.1570731672
Evento: XXXIX Simpósio Brasileiro de Telecomunicações e Processamento de Sinais (SBrT2021)
Keywords: Acoustic Scene Classification Audio Segmentation Multitaper-reassigned Spectrogram Time-frequency Entropies
Abstract
Acoustic Scene Classification (ASC) systems have great potential to transform existing embedded technologies. However, research on ASC has put little emphasis on solving the existing challenges in embedding ASC systems. In this paper, we focus on one of the problems associated with smaller ASC models: the generation of smaller yet highly-informative training datasets. To achieve this goal, we propose to employ the so-called multitapering-reassignment technique to generate high-resolution spectrograms from audio signals. These sharp time-frequency (TF) representations are used as inputs to a splitting method based on TF-related entropy metrics. We show via simulations that the datasets created through the proposed segmentation can successfully be used to train small convolutional neural networks (CNNs), which could be employed in embedded ASC applications.Download