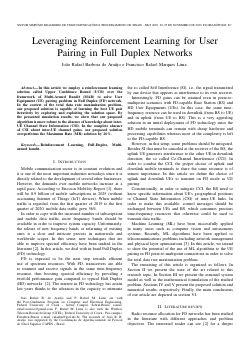
Leveraging Reinforcement Learning for User Pairing in Full Duplex Networks
João Rafael Barbosa de Araujo, Francisco Rafael Marques Lima
DOI: 10.14209/SBRT.2020.1570661436
Evento: XXXVIII Simpósio Brasileiro de Telecomunicações e Processamento de Sinais (SBrT2020)
Keywords: Reinforcement Learning Full-Duplex Multiarmed bandit
Abstract
In this article we employ a reinforcement learning solution called Upper Confidence Bound (UCB) over the framework of Multi-Armed Bandit (MAB) to solve User Equipment (UE) pairing problem in Full Duplex (FD) network. In the context of the total data rate maximization problem, our proposed solution is capable of learning the best UE pair iteratively by exploring and exploiting the solution space. By the presented simulation results, we show that our proposed algorithm is more robust to the absence of knowledge about inter-UE Channel State Information (CSI). In the complete absence of CSI about inter-UE channel gains, our proposed solution overperforms the Maximum Rate (MR) solution by 26%.Download