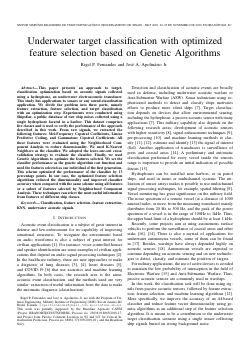
Underwater target classification with optimized feature selection based on Genetic Algorithms
Rigel Fernandes, José Antonio Apolinário Jr.
DOI: 10.14209/SBRT.2020.1570658075
Evento: XXXVIII Simpósio Brasileiro de Telecomunicações e Processamento de Sinais (SBrT2020)
Keywords:
Abstract
This paper presents an approach to target-classification optimization based on acoustic signals collected using a hydrophone, an underwater electroacoustic transducer. This study has applications to sonars or any sound-classification application. We divide the problem into three parts, namely feature extraction, feature selection, and target classification with an optimization step. Experiments were conducted using ShipsEar, a public database of raw ship noises collected using a single hydrophone located in a harbor. This dataset comprises five classes and is used to verify the performance of the approach described in this work. From raw signals, we extracted the following features: Mel-Frequency Cepstral Coefficients, Linear Predictive Coding, and Gammatone Cepstral Coefficients. All these features were evaluated using the Neighborhood Component Analysis to reduce dimensionality. We used K-Nearest Neighbors as the classifier. We adopted the leave-one-out cross-validation strategy to evaluate the classifier. Finally, we used Genetic Algorithms to optimize the features selected. We set the classifier performance as the genetic algorithm cost function and used the features selected as one individual of the first generation. This scheme optimized the performance of the classifier by 13 percentage points. In our case, the optimized feature selection algorithms reduced the dimensionality and improved classifier accuracy when compared with the same scheme using all features or a subset of features selected by Neighborhood Component Analysis. These techniques can select the most useful information from features of different ship classes.Download