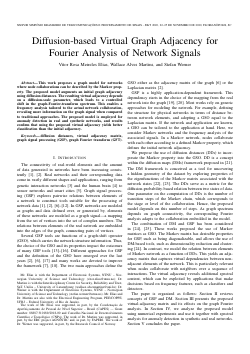
Diffusion-based Virtual Graph Adjacency for Fourier Analysis of Network Signals
Vitor R. M. Elias, Wallace A. Martins, Stefan Werner
DOI: 10.14209/SBRT.2020.1570649635
Evento: XXXVIII Simpósio Brasileiro de Telecomunicações e Processamento de Sinais (SBrT2020)
Keywords: diffusion distances virtual adjacency matrix graph signal processing (GSP) graph Fourier transform (GFT)
Abstract
This work proposes a graph model for networks where node collaborations can be described by the Markov property. The proposed model augments an initial graph adjacency using diffusion distances. The resulting virtual adjacency depends on a diffusion-scale parameter, which leads to a controlled shift in the graph-Fourier-transform spectrum. This enables a frequency analysis tailored to the actual network collaboration, revealing more information on the graph signal when compared to traditional approaches. The proposed model is employed for anomaly detection in real and synthetic networks, and results confirm that using the proposed virtual adjacency yields better classification than the initial adjacency.Download