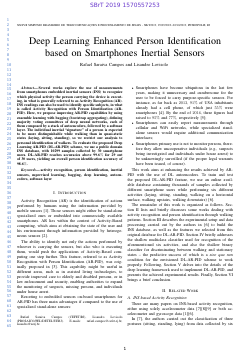
Deep Learning Enhanced Person Identification based on Smartphones Inertial Sensors
Rafael Saraiva Campos, Lisandro Lovisolo
DOI: 10.14209/sbrt.2019.1570557253
Evento: XXXVII Simpósio Brasileiro de Telecomunicações e Processamento de Sinais (SBrT2019)
Keywords:
Abstract
Several works explore the use of measurements from smartphones embedded inertial sensors (INS) to recognize which physical activity the person carrying the device is executing, in what is generally referred to as Activity Recognition (AR). INS readings can also be used to identify specific subjects, in what is called Activity Recognition with Person Identification (ARPID). Here, we propose improving AR-PID capabilities by using ensemble learning with bagging (bootstrap aggregating), defining majority voting committees of deep neural networks, each of them composed by a stack of autoencoders, followed by a softmax layer. The individual inertial "signature" of a person is expected to be more distinguishable while walking than in quasi-static states (laying, sitting, standing), so we restrict our analysis to personal identification of walkers. To evaluate the proposed Deep Learning AR-PID (DL-AR-PID) scheme, we use a public domain INS database, with 10299 samples collected by 30 smartphone users. DL-AR-PID reaches accuracies above 99.6% for 29 out of 30 users, yielding an overall person identification accuracy of 98.6%.Download