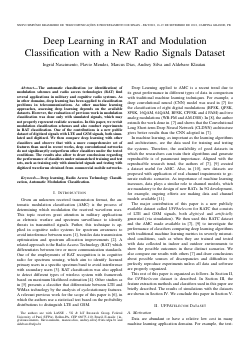
Deep Learning in RAT and Modulation Classification with a New Radio Signals Dataset
Ingrid Nascimento, Flavio Mendes, Marcus Dias, Andrey Silva, Aldebaro Klautau
DOI: 10.14209/sbrt.2018.144
Evento: XXXVI Simpósio Brasileiro de Telecomunicações e Processamento de Sinais (SBrT2018)
Keywords: Deep learning Radio Access Technology Classification Automatic Modulation Classification
Abstract
The automatic classification (or identification) of modulation schemes and radio access technologies (RAT) find several applications in military and cognitive radio systems. As in other domains, deep learning has been applied to classification problems in telecommunications. As other machine learning approaches, assessing deep learning depends on the available datasets. However, the evaluation of previous work in modulation classification was done only with simulated signals, which may not properly represent realistic scenarios. In this paper, we revisit modulation classification schemes and also conduct experiments in RAT classification. One of the contributions is a new public dataset of digitized signals with LTE and GSM signals, both simulated and digitized. We then compare deep learning with other classifiers and observe that with a more comprehensive set of features than used in recent works, deep convolutional networks do not significanytly outperform other classifiers under the tested conditions. The results also allow to draw conclusions regarding the performance of classifiers under mismatched training and test sets, such as training only with simulated signals and testing with digitized waveforms obtained from commercial mobile networks.Download