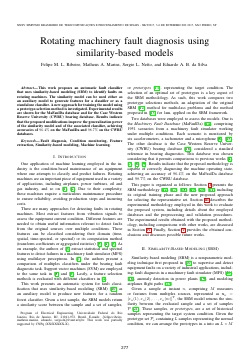
Rotating machinery fault diagnosis using similarity-based models
Felipe M. L. Ribeiro, Matheus A. Marins, Sergio L. Netto, Eduardo A. B. da Silva
DOI: 10.14209/sbrt.2017.133
Evento: XXXV Simpósio Brasileiro de Telecomunicações e Processamento de Sinais (SBrT2017)
Keywords: Fault diagnosis Condition monitoring Feature extraction Similarity-based modeling Machine learning
Abstract
This work proposes an automatic fault classifier that uses similarity-based modeling (SBM) to identify faults on rotating machines. The similarity model can be used either as an auxiliary model to generate features for a classifier or as a standalone classifier. A new approach for training the model using a prototype-selection method is investigated. Experimental results are shown for the MaFaulDa database and for the Case Western Reserve University (CWRU) bearing database. Results indicate that the proposed modifications improve the generalization power of the similarity model and of the associated classifier, achieving accuracies of 96.4% on the MaFaulDa and 98.7% on the CWRU databases.Download