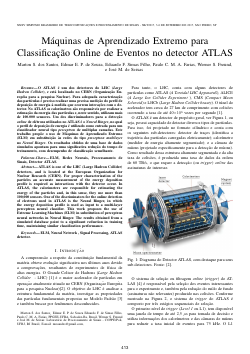
Máquinas de Aprendizado Extremo para Classificação Online de Eventos no detector ATLAS
Marton S. dos Santos, Edmar E. P. de Souza, Eduardo F. Simas Filho, Paulo C. M. A. Farias, Werner S. Freund, José M. de Seixas
DOI: 10.14209/sbrt.2017.103
Evento: XXXV Simpósio Brasileiro de Telecomunicações e Processamento de Sinais (SBrT2017)
Keywords: ELM Neural Network Signal Processing ATLAS detector
Abstract
ATLAS is one of the LHC (Large Hadron Collider) detectors, and is located at the European Organization for Nuclear Research (CERN). For proper characterization of the particles an accurate measurement of the energy deposition profile is required as interactions with the detector occur. In ATLAS, the calorimeters are responsible for estimating the energy of the particles and, in this sense, they use more than 100,000 sensors. One of the discriminators for the online detection of electrons used in ATLAS is the Neural Ringer, in which the energy deposition profile is used as input to a multi-layer perceptron neural classifier. This work proposes the use of Extreme Learning Machines (ELM) in substitution of perceptron neural networks in Neural Ringer. The results obtained from a simulated database point to a significant reduction in training time, maintaining similar classification performance.Download