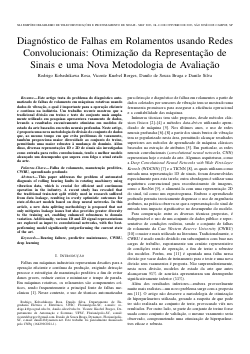
Diagnóstico de Falhas em Rolamentos usando Redes Convolucionais: Otimização da Representação de Sinais e uma Nova Metodologia de Avaliação
Rodrigo K Rosa, Vicente K Borges, Danilo de S. Braga, Danilo Silva
DOI: 10.14209/sbrt.2023.1570915812
Evento: XLI Simpósio Brasileiro de Telecomunicações e Processamento de Sinais (SBrT2023)
Keywords: Bearing failure predictive maintenance CWRU deep learning
Abstract
This paper addresses the problem of automated diagnosis of rolling bearing faults in rotating machinery using vibration data, which is crucial for efficient and continuous operation in the industry. A recent study has revealed that the traditional train-test split widely used in research suffered from data leakage, resulting in overly optimistic outcomes for state-of-the-art models based on deep neural networks. In this article, a new data splitting methodology is proposed that not only mitigates leakage issues but also provides greater diversity to the training set, enabling enhanced robustness to domain variation. Additionally, various 1D and 2D signal representations are explored as input to convolutional networks, with the best-performing model significantly outperforming the current state of the art.Download