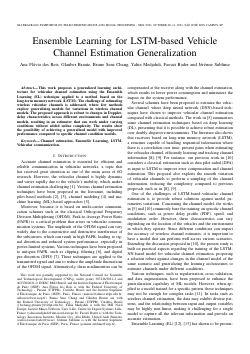
Ensemble Learning for LSTM-based Vehicle Channel Estimation Generalization
Ana Flávia Reis, Bruno Chang, Glauber Brante, Yahia Medjahdi, Faouzi Bader, Jérémie Sublime
DOI: 10.14209/sbrt.2023.1570908283
Evento: XLI Simpósio Brasileiro de Telecomunicações e Processamento de Sinais (SBrT2023)
Keywords: Channel estimation Ensemble Learning LSTM Vehicular communication
Abstract
This work proposes a generalized learning architecture for vehicular channel estimation using the Ensemble Learning (EL) technique applied to a method based on the long-term memory network (LSTM). The challenge of estimating wireless vehicular channels is addressed, where few methods explore generalizing models for variations in wireless channel models. The proposed approach is robust to changes in Doppler-delay characteristics across different environments and channel models, resulting in an estimator that can work under varying conditions without added online complexity. The results show the possibility of achieving a generalized model with improved performance compared to specific channel condition models.Download