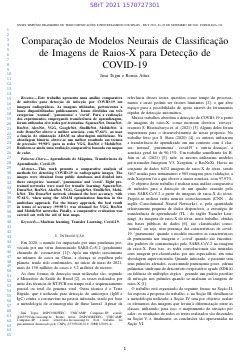
Comparação de Modelos Neurais de Classificação de Imagens de Raios-X para Detecção de COVID-19
Jimi Togni, Romis Ribeiro Attux
DOI: 10.14209/sbrt.2021.1570727301
Evento: XXXIX Simpósio Brasileiro de Telecomunicações e Processamento de Sinais (SBrT2021)
Keywords: Aprendizado de Máquina Deep Learning COVID-19 Transferência de Aprendizado
Abstract
This work presents a comparative analysis of methods for detecting COVID-19 infection in radiographic images. The images used, belonging to publicly available databases, were divided into three categories: 'normal', 'pneumonia' and 'covid'. For carrying out the experiments, based on the technique known as transfer of learning, eight pre-trained networks were used, all commonly used for image classification and recognition: SqueezeNet, DenseNet, ResNet, AlexNet, VGG, GoogleNet, ShuffleNet, MobileNet. We opted to use these networks because they are widely used methodologies and cited in recent academic works on computer vision, detection and classification of images. As a result, it was observed that the DenseNet network obtained the best accuracy, with 97.64%, when using the ADAM optimization function in the multiclass approach. The binary approach, in turn, obtained the best result in terms of accuracy: 99.98% on the VGG, AlexNet and MobileNet networks. Finally, a comparative assessment is carried out, supported by the inspection of heat maps.Download