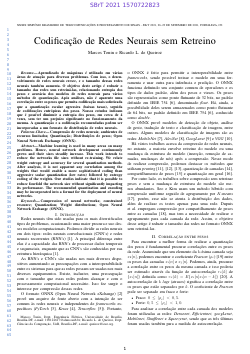
Codificação de Redes Neurais sem Retreino
Marcos Vinicius Tonin, Ricardo L de Queiroz
DOI: 10.14209/sbrt.2021.1570722823
Evento: XXXIX Simpósio Brasileiro de Telecomunicações e Processamento de Sinais (SBrT2021)
Keywords: Compressão de redes neurais ambientes de recursos limitados Distribuições de pesos Open Neural Network Exchange (ONNX)
Abstract
Machine learning is used in many areas on many problems. Hence, neural network development continuously grows and their sizes steadily increase. This work intends to reduce the networks file sizes without re-training. We relate weight entropy and accuracy for several quantization methods. It is not apparent any correlation among the weights that would enable a more sophisticated coding than aggressive scalar quantization followed by entropy coding of the weights. Our studies indicate that it is possible to reduce fourfold the network size without significantly impacting its performance. The recommended quantization and encoding may be incorporated into a format for the deployment of neural networks.Download