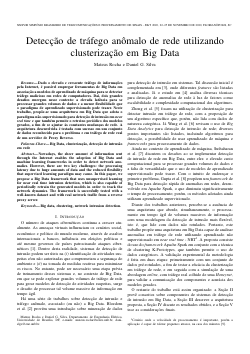
Detecção de tráfego anômalo de rede utilizando clusterização em Big Data
Mateus Rocha, Daniel G Silva
DOI: 10.14209/SBRT.2020.1570658430
Evento: XXXVIII Simpósio Brasileiro de Telecomunicações e Processamento de Sinais (SBrT2020)
Keywords: Big Data clustering network intrusion detection
Abstract
Nowadays, the sheer amount of information sent through the Internet enables the adoption of Big Data and machine learning frameworks in order to detect network anomalies. However, there are two key challenges: the processing latency due to huge amounts of data and the reduced flexibility that supervised learning paradigm may cause. In this paper, we propose a Big Data framework that uses unsupervised learning for near real time intrusion detection, which is also capable of periodically retrain the generated models in order to track the network dynamics. The framework is succesfully tested with a well-known dataset and with real network traffic from a reverse proxy server.Download