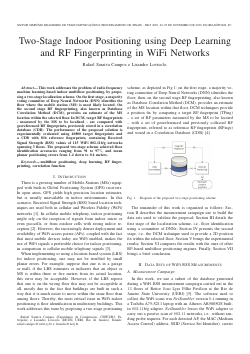
Two-Stage Indoor Positioning using Deep Learning and RF Fingerprinting in WiFi Networks
Rafael Saraiva Campos, Lisandro Lovisolo
DOI: 10.14209/SBRT.2020.1570646073
Evento: XXXVIII Simpósio Brasileiro de Telecomunicações e Processamento de Sinais (SBrT2020)
Keywords: multifloor positioning deep learning RF fingerprinting correlation function
Abstract
This work addresses the problem of radio frequency machine learning-based indoor multifloor positioning, by proposing a two-stage localization scheme. On the first stage, a majority voting committee of Deep Neural Networks (DNN) identifies the floor where the mobile station (MS) is most likely located. On the second stage RF fingerprinting, also known as Database Correlation Method (DCM), provides an estimate of the MS location within the selected floor. In DCM, target RF fingerprints - measured by the MS to be localized - are compared with georeferenced RF fingerprints, previously stored in a correlation database (CDB). The performance of the proposed solution is experimentally evaluated using 40800 target fingerprints and a CDB with 816 reference fingerprints, containing Received Signal Strength (RSS) values of 115 WiFi 802.11b/g networks spanning 3 floors. The proposed two-stage scheme achieved floor identification accuracies ranging from 91 to 97%, and mean planar positioning errors from 1.4 down to 0.4 meters.Download